What Can 11,000 Proteins In Our Blood Tell Us?
The beginnings of a quiet revolution in high-throughput proteomics and A.I.
Last week in Science, I published an essay entitled “The revolution in high-throughput proteomics and AI.” But in the few weeks since I wrote it, there’s been much more to add to the story. Let me first summarize the background and we’ll build from there.
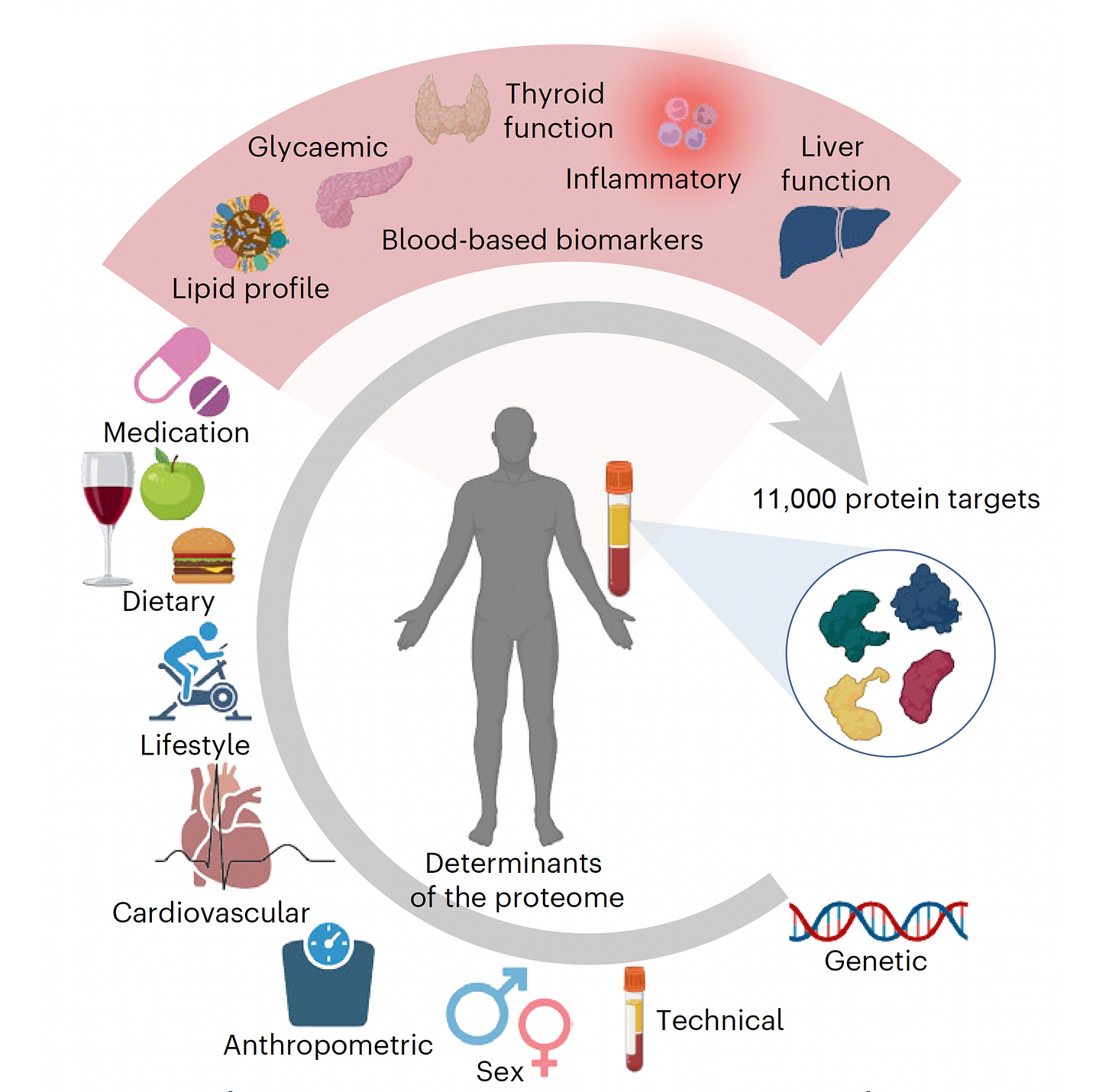
Background
The recent capability to measure thousands of plasma proteins from a tiny blood sample has provided a new dimension of expansive data that can advance our understanding of human health. For example, the company SomaLogic has developed the means to measure 11,000 proteins and Thermo Fisher’s Olink assays over 5,400 proteins from as little as 2 μl. (This is real; not Theranos!] When these rich data are integrated with other layers of information from large patient cohorts, such as the UK Biobank’s genetic, health, and lifestyle information from half a million participants, we get new insights about the underpinnings of disease, the aging process, and the potential ability to forecast an individual’s health trajectory.
Proteins in Contrast to Genome Variants
For over a decade, polygenic risk scores that reflect the likelihood of a certain genetic trait manifesting as a disease have been developed that partition risk for most common diseases. This approach has been validated across groups of people from different ancestries and is now beginning to be used for patient guidance. These risk scores are typically based on the presence of hundreds of common (present in >5% of the population) single-nucleotide polymorphisms (SNPs). But we know that the risk for disease is not just reflected by common DNA sequence variants. There are rare and ultra-rare genomic variants that are not factored in to calculating risk, such as the insertion-deletion (indel) of nucleotides in DNA that generate mutations, or changes in the arrangement of DNA (rather than just single nucleotide changes) that cause structural variations. Moreover, there is variability in our proteomic, metabolomic, and epigenomic profiles as well as in our microbiome, immunome, and exposomes (environmental exposures). What was envisioned in 2000 when the human genome sequence draft was announced as our “operating instructions” turns out to be a critical, but incomplete, layer of information about what makes us tick—and our susceptibility to various medical conditions.
In contrast to about 20,000 protein-coding genes in the human genome, there are over 100,000 different proteins and, as a result of alternative splicing, hundreds of thousands of protein isoforms (variants) in the human body. Being able to assay for a substantial fraction of these is the basis of hypothesis-free research—that is, efforts to gather data and blindly seek patterns as opposed to hypothesizing that certain patterns exist and then seeking them out. This approach, along with machine learning analytics, has led to a revolution in understanding the foundations of disease.
Organ Clocks
Several recent high-throughput proteomic studies are illuminating at both the organ and body-wide level. Three studies have addressed organ-specific protein dynamics. Oh and colleagues culled data from five groups of individuals (three with healthy participants, two with Alzheimer’s disease patients) assembled from 5676 adults to assess nearly 5000 plasma proteins (and with a 5-year follow-up). Using machine learning models, proteins that are specific to 11 organs were identified and an “organ age gap” was derived by comparing biological with chronological age. About one in five individuals were hyper-agers for at least one organ (thus, an organ was physiologically and functionally much older than the person's chronological age), and 2% for multiple organs. For each of the 11 organs, the age gap was associated with an increased risk of mortality.
Two subsequent studies took this finding further. In a preprint reported by Gladyshev and colleagues, about 3000 plasma proteins were assessed in 53,000 UK Biobank participants. With machine learning, seven organ-specific aging clocks were defined. Lifestyle factors such as smoking were associated with an increased pace of aging for all seven organs, as was alcohol for intestinal aging. Organ aging also was associated with many other factors including various foods, medications, and occupations. A third study, again by Oh and colleagues, also in preprint form, assayed 3000 plasma proteins in 44,000 UK Biobank participants, and further validated the 11 organ-specific aging clocks. Postmenopausal estrogen replacement was associated with slower aging across most organs, in contrast to smoking and alcohol. A notable finding was that slow brain or immune system aging was associated with improved survival over an extended 15-year follow-up.
More on this topic in a previous Ground Truths post.
Body-Wide Aging Clocks
Turning to body-wide high throughput proteomics, Argentieri and colleagues analyzed nearly 3000 plasma proteins in over 45,000 UK Biobank participants, and did further cross-validation in a China biobank of about 4000 participants, and one in Finland of nearly 2000 individuals, with at least 11 years of follow-up for each cohort. A cluster of 204 proteins not only predicted chronological age accurately, but was also associated with 18 chronic diseases, including four common cancers, multimorbidity, and all-cause mortality (death from any cause). Rapid proteomic clock agers (individuals whose protein pattern indicated aging at an abnormally fast pace) were at higher risk of Alzheimer’s disease, whereas among slow proteomic clock agers (in the bottom decile), less than 1% developed Alzheimer’s disease.
Two reports of body-wide proteomics with artificial intelligence (AI) models by Carrasco-Zanini and colleagues widened the field of prediction for diseases. In the EPIC-Norfolk cohort of over 25,000 participants, about 3000 plasma proteins were assayed and associated with risk of 13 diseases and all-cause mortality. More broadly, in over 41,000 individuals from the UK Biobank, through assessing the same plasma proteins and integrating the data with electronic health records, there were varying levels of predictability for risk for 52 of 218 common and rare diseases.
Proteomic Peaks Along the Lifespan Suggest Aging Isn’t A Linear Process
With respect to aging, proteomic studies have indicated that it is not a linear process. In 2019, Lehallier and colleagues found, among over 4000 people aged 18 to 95 years, that across almost 3000 proteins, there were three proteomic peaks (highest expression of proteins) during our life-span at around age 35, 60, and 80 years. More recently, a multi-omic study that assessed over 300 proteins, but was limited to an upper age of 75 years (and with a short follow-up of1.7 years), identified proteomic peaks at ages 44 and 61.
Williams et al. studied the predictive potential of approximately 5,000 proteins in nearly 17,0000 individuals and found proteins that were strongly linked with risk of cardiovascular disease, diabetes, and metabolic-associated fatty liver disease. Other high-throughput proteomic studies have focused on the risk of dementia in healthy individuals and on Alzheimer’s disease that indexed to a person's expressed variant of the apolipoprotein E (APOE) gene.
Summary Table of the Above 11 Studies
Establishing Cause and Effect Proteins
Lars Lind and colleagues recently published the proteomic data (O-link assay, 2,919 proteins) data from over 52,000 UK Biobank participants and replicated their findings in the China Kadoorie biobank.Using the protein data along with Mendelian Randomization (MR) from the genomic data (for a nice explanation on MR, see the transcript of my podcast with Pradeep Natarajan, starting at 7:13), they found 3 causal proteins for cardiovascular outcomes (heart attack, ischemic stroke, heart failure) that likely fulfilled a cause-and-effect relationship, including FURIN, FGF5, and PROCR. None of these proteins are currently targets in clinical trials, but all have potential to be druggable for prevention of adverse cardiovascular outcomes.
Julia Carrasco-Zanani and colleagues assessed 4,775 plasma proteins in about 8,000 healthy participants integrated with genomic data, again setting up Mendelian Randomization analysis, establishing causal inference, identifying shifts in the plasma proteome—biological drivers—from actionable lifestyle/behavioral modifiable risk factors. Of over a hundred potential causal drivers, one protein example was COL6A3 (Figure below) that appeared to mediating reduced kidney function in individuals with heart disease.
Here are the disease-associated proteins per major biological contributor to the plasma proteome. While also identifying putative drug targets, this report homed in on identifying modifiable risk factors, many of which are novel. Few dietary factors were found in this interrogation of modifiable protein drivers.
Proteomics and Diets
That turned out to be the focus of another new report by Kai Zhu a colleagues who used the data from nearly 22,000 UK Biobank participants with ~11 years of follow-up, who had assays of over 2,900 O-link plasma proteins. The 2 questions addressed were: (1) do 8 healthy diets have proteomic signatures?, and (2) how were these proteomic signatures associated with major disease outcomes and life expectancy?
The healthy diets and their proteomic signatures were associated with reduced all-cause mortality, Type 2 diabetes, dementia, cancer, chronic kidney and lung diseases. The graph below shows the estimated added life expectancy for a participant age 45 years by diet and by proteomic signature (highest versus lowest tenth percentiles). The healthful plant-based index diet (hPDI) Y-axis is different reflecting the impact of its proteomic signature. Specific proteins were identified as mediators, such as FSTL3, interleukin-18 receptor 1, and hepatocyte growth factor.
Below you can see the individual foods within the 8 diets, relationship with the 7 outcomes at left. Associated reduced risk is in blue, increased risk in red. This study is noteworthy because most dietary studies rely of food diaries and the memory of participants. The tracking with a proteomic signature could provide a more objective assessment of adherence to a particular diet.
Bottom Line
Collectively, these studies highlight the new and extraordinary ability to assay and learn about a broad swath of our plasma proteins. The work to date already has enhanced our understanding of the human aging process, identified many organ-specific changes and how they may be favorably modulated, raised the potential of using proteomic scores for assessing risk of various diseases, identified novel protein drivers that are modifiable, and a complementary signature for traditional dietary assessment. Note that the studies reviewed here “only” assessed less than 5,000 proteins, but that number is rapidly increasing, now up to 11,000 and will undoubtedly keep increasing.
Of course, proteomics represents only one layer of data that can, with the use of multimodal AI analytics, be orthogonally integrated with the electronic health record, genomic risk, epigenetic clocks, biomarkers of inflammation, the gut microbiome, the immune system function, and environmental exposures. A limitation of the high-throughput proteomic assessment is the expense, ranging from $500 to $1000 per individual. But validated protein panel subsets for specific risk assessment may lead to much lower costs. Not to be missed at this juncture is that we indeed are seeing an exciting development of the proteomics field and AI, which will continue to build and ultimately find its place in routine medical care.
**********************************************
Thanks for reading and subscribing to Ground Truths.
If you found this interesting please share it!
That makes the work involved in putting these together especially worthwhile.
All content on Ground Truths—its newsletters, analyses, and podcasts, are free, open-access.
Paid subscriptions are voluntary and all proceeds from them go to support Scripps Research. They do allow for posting comments and questions, which I do my best to respond to. Many thanks to those who have contributed—they have greatly helped fund our summer internship programs for the past two years.
Thank you Eric for the well written article on a blood protein analyses correlated with Biobank data. Let’s hope that the accelerate disease prevention and drug discovery. When will these analyses supplant CBC, Chem, Lipid panels, etc?
Thank you for this article! So much exciting information to follow up with for the future, and the potential positive impacts on human health guidelines and preventative recommendations.