A New Precedent—A.I. Gets the "American Nobel" Prize in Medicine
AlphaFold was the first A.I. to receive the Lasker Award since these began in 1945
Not long ago scientists might spend 2 or 3 years to define the 3-dimensional structure of a protein, relying on many advanced tools like cryogenic electron microscopy and crystallography. It was painstaking work and only a relatively limited number of proteins could be approached, many unsuccessfully. Now that can be done for nearly all proteins in a matter of minutes, thanks to advances in A.I. Even new proteins, not existing in nature, never previously conceived, can now be created.
The work in life science to predict 3D protein structure from amino acid sequence traces back to the 1960s, and in more recent times leading to the CASP (Critical Assessment of Structure Prediction) biannual meetings to provide objective assessment of the progress. At the CASP 2018 meeting the DeepMind A.I. company group, led by Demis Hassabis and John Jumper, introduced AlphaFold, which relied on deep learning (a convolutional neural network) and a biophysical approach to achieve a significant jump in accuracy (58.9 out of 100), at the median accuracy level of 6.6 angstroms (distance between the atoms in the proposed structure vs actual positions). But that was superseded at the next CASP meeting in 2020 with AlphaFold2, based on a transformer model, with accuracy at 92.4 (out of 100) at 1.5 angstroms (the width of an atom), which defines atomic accuracy. This extraordinary A.I advance for accurately predicting 3D protein structure from 1D amino acid sequence, a holy grail in life science, was widely recognized by the science community, as reflected by designation as the Breakthrough of the Year for 2021 in both Nature Methods and Science.
The Evolution From AlphaFold1 to AlphaFold2
I’ve summarized the transition from AlphaFold1 to 2 in the graphic below, with an extraordinary acceleration of progress in predicting 3D protein structure from the Nature paper in 2021 to predictions approaching the complete protein universe in 2022. The A.I. tool was made open-source and is one of several A.I. transformer models inspired by AlphaFold for predicting 3D protein structure, that includes RoseTTAFold from David Baker and colleagues at the University of Washington, ESMFold by Meta, OmegaFold and others. These latter transformer protein learning models added more tracks in their neural architecture beyond AlphaFold2’s two-track transformer (called Evoformer) for 3D space coordination [known as SE(3)-transformer]. At the time of the July 2002 DeepMind release of “the entire protein universe” , when the data resource was expanded to over 200 million protein structures (which includes the >20,000 human proteins), I was quoted as this being “the singular and momentous advance in life science that demonstrates the power of A.I.”
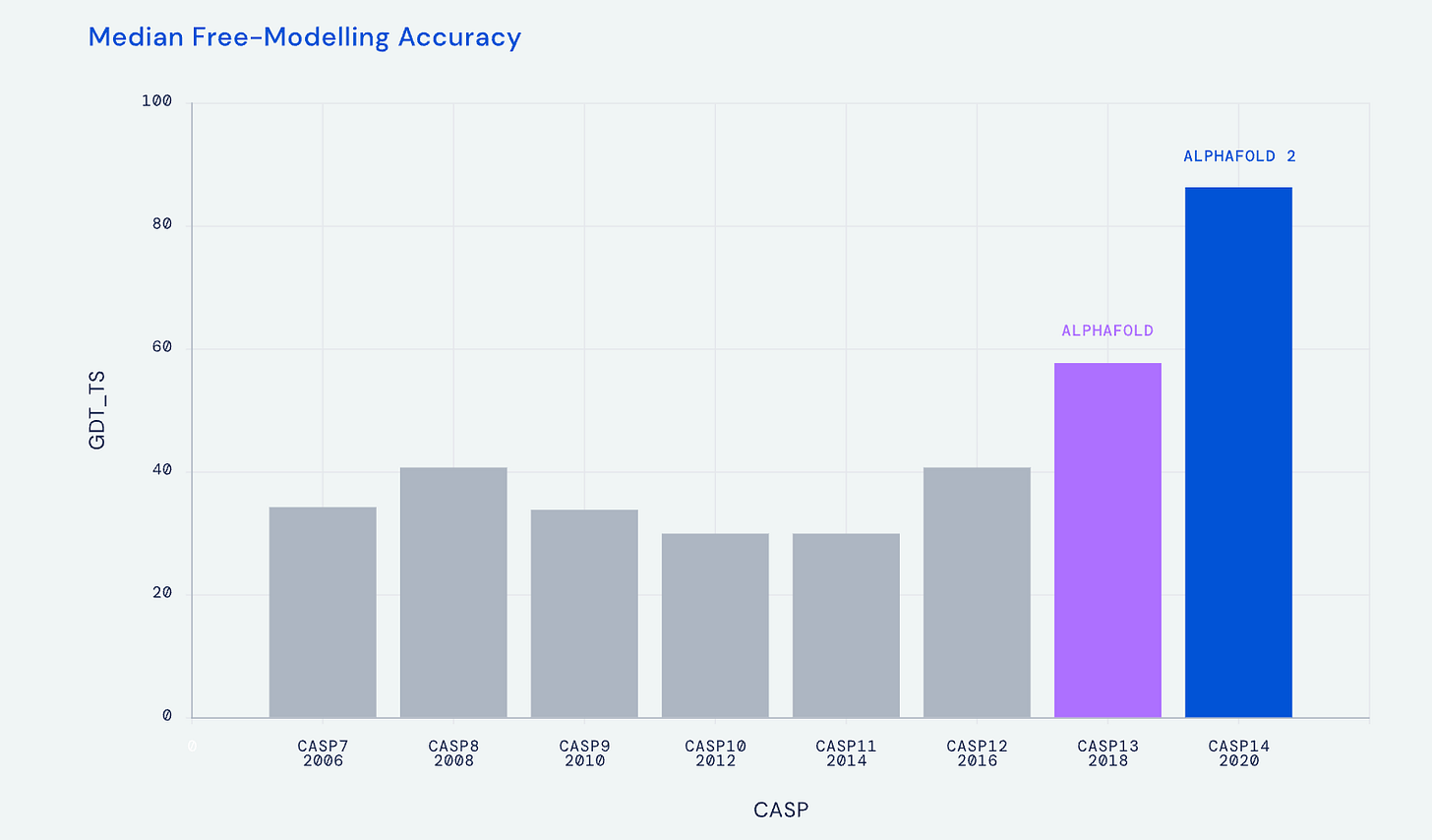
On September 21, 2023, the annual Albert Lasker Foundation Basic Medical Research Award went to AlphaFold, and specifically to Demis Hassabis and John Jumper, for their “brilliant ideas, intensive efforts and supreme engineering.”
The Lasker Foundation has been giving these awards each year since 1945 and they are often predictive of future Nobel Prize laureates: 95 Lasker awardees have received the Nobel prize. Lasker Awards are considered the highest medical-scientific award in the United States. Accordingly, the Laskers are known as the “American Nobels.” On Monday, October 2nd, the Nobel Prize in Physiology or Medicine was awarded to Kariko and Weissman, for their work that paved the way for mRNA vaccines. They were previous Lasker Award and Breakthrough Prize winners, like Hassabis and Jumper. It is certainly possible that AlphaFold will be a future recipient for the Nobel Prize.
In the long history of the Lasker Awards—78 years—there has never been one for A.I. Sure, it was a group of humans that guided AlphaFold2 (now simply known as AlphaFold, which omits its early history and predecessor) to its smashing success. But, as Demis Hassabis has noted, “The fascinating thing about A.I. in general is that it’s kind of a black box. But ultimately it seems like it’s learning actual rules about the natural world.” While this is generally true for many A.I. deep neural networks, it is especially the case for transformer models, they aren’t explainable—we don’t know how they work. I think it’s a fair assertion that AlphaFold wouldn’t have been possible without transformer A.I. models —also the basis for large language models (LLMs) like ChatGPT and GPT-4. (And yes, just like LLMs, AlphaFold can hallucinate protein structures.) It was really the combination of human ingenuity and the “black box” of a transformer model that accounts for the Lasker Award this year! However, with this precedent, the humans only recognized other humans.
AlphaFold Dividends
Now over 1.2 million researchers from more than 200 countries have accessed AlphaFold to view millions of structures. The transformer protein learning models are catalyzing breakthroughs in life science, and I’ll just highlight 3 major biology advances here that would not have been possible without AlphaFold.
The nuclear pore complex, the largest molecular machine in human cells (in every cell that has a nucleus) with more than 1,000 protein subunits, connects everything from cytoplasm to nucleus bidirectionally, and is considered the world’s hardest, giant jigsaw puzzle. Thanks to AlphaFold, it finally had its 3D structure cracked at near atomic level resolution (here and here and here) with a dedicated issue of Science in 2022.
In March this year, Feng Zhang and colleagues used AlphaFold to design a “molecular syringe” for programmable protein delivery into cells. This has broad potential applications for the treatment of cancer, design and delivery of vaccines, and gene therapy.
More recently,in August, Gu et al published on the midnolin pathway, which is a bypass, alternative path for degradation of nuclear proteins.
Of course, there is intense interest for the use of AlphaFold and protein learning models for drug discovery. Indeed, Hassabis formed a drug discovery company known as Isomorphic Labs. This is a big topic in itself and worthy of a future whole Ground Truths edition. Suffice it to say here that A.I. is transforming and markedly accelerating drug discovery, as shown below for protein drugs, and there are many A.I.-derived drugs that have entered clinical trials.
AlphaFold Imperfections
It is critical to point out that AlphaFold and the transformer protein learning models are far from perfect, well outside the confidence predictions of protein-structure, and there are still many unresolved. Moving to small molecule interactions with proteins is a problem, as Masha Karelina asserted: “docking to AlphaFold models is much less accurate than to protein structures that are experimentally determined.” As I wrote in a recent Ground Truths about my structural biologist colleague at Scripps Research, Jane Dyson, for sick, disordered proteins: “the A.I.’s predictions are mostly garbage. It’s not a revolution that puts all of our scientists out of business.” AlphaFold doesn’t explain why proteins fold the way they do. It can have difficulties with alternative splicing. The list goes on.
Transformer A.I. Beyond Protein Prediction
Missense Mutations
AlphaMissense, by Google DeepMind researchers, Jun Cheng, Ziga Avsec, and colleagues, was published last month —19 September 2023—using a transformer model derived from AlphaFold (Evoformer) to predict all 71 million human missense variants, as categorized below.
RNA
While it has been characterized as a “grand challenge,” in 2021 deep learning was used to predict 3D RNA structure with ARES (Atomic Rotationally Equivariant Scorer) which outperformed all prior models. Last week, the team at Deep Genomics preprint published “BigRNA,” a foundation model for multiple predictions including protein and micro-RNA binding sites and the effect of variants. This advance was enabled by a multi-head transformer model.
Designer Proteins
In July, David Baker and his group at UW published RF Diffusion, built on their RoseTTAfold transformer model, which enables design of complex proteins structures, and performed far better than their previous tool, interestingly called hallucination. RF Diffusion is making protein structures that bind strongly to key proteins in cancer (such as p53), autoimmune diseases, antibiotic-resistant infections and many others, with certain specifications such as length and folds. It’s still a work in progress, since less than 1 in 5 of the designed proteins binding strongly to the target, but it’s an exciting new path that transformer models have paved.
Single-Cell Research and Gene Networks
Transformer models are being applied to single-cell work, the basis of spatial biology, an important topic I recently reviewed (“Spatial Biology is Lighting It Up”). As one example, Geneformer was published in Nature May 2023, pretrained from ~30 million single-cell transcriptomics, making predictions about the network of interacting genes.
Summing Up: Transformer A.I. Models and the Nobel Prize
While we have been absorbed by and fascinated with large language models with text and more recently extended multimodally with images, video, and speech, generative A.I. has now been shown to transform life science, building molecules atom by atom, bond by bond. In all the examples provided above, this remarkable progress was enabled by transformer models. The old (2011) proclamation that “software is eating the world” could be adapted here as transformer models are eating—profoundly impacting—life science. The jump from prior deep learning algorithms, particularly convolutional neural networks, to transformer models, has led to a series of breakthroughs with AlphaFold as the archetype.
Which brings us to the Nobel Prize. This week, in the Wall Street Journal, David Oshinsky wrote a long piece about the reasons that the Nobels need a makeover. Conspicuously absent was the mention of A.I. From the new precedent AlphaFold Lasker Award, we now know that it’s no longer solely humans who are responsible for a discovery. Hassabis and Jumper had a big team at DeepMind to help unravel the 3D protein universe, but one of those helpers was not human—it was a transformer model. I’m not trying to make the case that the A.I. model here is like the invaluable but Nobel-unrecognized contributions of Rosalind Franklin, but the machine played a critical, essential role in the invention. So much so, that we don’t fully understand how it was accomplished. As Al Gore foreshadowed with me during our recent podcast, “there is an unpredictable unquantifiable risk that we might no longer be the apex lifeform on this planet.” We obviously are not there yet, but it does seem that in the case of the Lasker Award, the transformer A.I. model, along with the brilliant brainchild of Hassabis and Jumper, should have received some credit. Many will argue justifiably that transformer models were created and refined by humans and they got all the attention they need (yes, it’s play on their 2017 discovery title). Yet it may be hard for humans to accept and recognize the fundamental role of generative A.I. for advances in science, just as we are struggling with how to deal with any LLM input for scholarly publications or other creative work. Perhaps a Nobel Prize in the future will show the way.
Thanks for reading and subscribing to Ground Truths!
If you found this informative or stimulating, please share with your network.
This essay was written by a human without any contribution of a transformer model.
All proceeds from Ground Truths go to Scripps Research.
I'm quite concerned about the label "A.I.".
The extraordinary achievements made in identifying protein folding configurations were the results of efforts made by extremely intelligent coders who designed a PROGRAM which is neither artificial nor intelligent.
The analogous model would be semiconductors. The fact that quantum computing works and tunneling occurs in non-intuitive ways makes neither the electron nor the computer intelligent.
This is not a mere question of semantics. Turing notwithstanding, the use of such monikers creates a perceived reality which has not been shown to exist.
Awesome. I hope they continue to honor the wee human geniuses who created these AI techs. We will someday be the ancient and fragile gods who spawned the creatures that colonize the galaxy… assuming we can get through this climate crisis and other existential threats, and the return of 1980’s style clothing.