When I think of digital biology, I think of Patrick Hsu—he’s the prototype, a rarified talent in both life and computer science, who recently led the team that discovered bridge RNAs, what may be considered CRISPR 3.0 for genome editing, and is building new generative A.I. models for life science. You might call them LLLMs-large language of life models. He is Co-Founder and a Core Investigator of the Arc Institute and Assistant Professor of Bioengineering and Deb Faculty Fellow at the University of California, Berkeley.
Above is a brief snippet of our conversation. Full videos of all Ground Truths podcasts can be seen on YouTube here. The audios are also available on Apple and Spotify.
Here’s the transcript with link to the audio and external links to relevant papers and things we discussed.
Eric Topol (00:06):
Well hello, it's Eric Topol with Ground Truths and I'm really delighted to have with me today Patrick Hsu. Patrick is a co-founder and core investigator at the Arc Institute and he is also on the faculty at the University of California Berkeley. And he has been lighting things up in the world of genome editing and AI and we have a lot to talk about. So welcome, Patrick.
Patrick Hsu (00:29):
Thanks so much. I'm looking forward to it. Appreciate you having me on, Eric.
The Arc Institute
Eric Topol (00:33):
Well, the first thing I'd like to get into, because you're into so many important things, but one that stands out of course is this Arc Institute with Patrick Collison who I guess if you can tell us a bit about how you two young guys got to meet and developed something that's really quite unique that I think brings together investigators at Stanford, UCSF, and Berkeley. Is that right? So maybe you can give us the skinny about you and Patrick and how all this got going.
Patrick Hsu (01:05):
Yeah, sure. That sounds great. So we started Arc with Patrick C and with Silvana Konermann, a longtime colleague and chemistry faculty at Stanford about three years ago now, though we've been physically operational just over two years and we're an independent research institute working at the interface of biomedical science and machine learning. And we have a few different aspects of our model, but our overall mission is to understand and treat complex human diseases. And we have three pillars to our model. We have this PI driven side of the house where we centrally fund our investigators so that they don't have to write grants and work on their very best ideas. We have a technical staff side of the house more like you'd see in a frontier AI lab or in biotech industry where we have professional teams of R&D scientists working cross-functionally on higher level organizational wide goals that we call our institute initiatives.
(02:05):
One focused on Alzheimer's disease experimentally and one that we call a virtual cell initiative to simulate human biology with AI foundation models. And our third pillar over time is to have things not just end up as academic papers, but really get things out into the real world as products or as medicines that can actually help patients on the translational side. And so, we thought that some really important scientific programs could be unlocked by enabling new organizational models and we are experimenting at the institutional scale with how we can better organize and incentivize and support scientists to reach these long-term capability breakthroughs.
Patrick, Patrick and Silvana
Eric Topol (02:52):
So the two Patrick’s. How did you, one Patrick I guess is a multi-billionaire from Stripe and then there's you who I suspect maybe not quite as wealthy as the other Patrick, how did you guys come together to do this extraordinary thing?
Patrick Hsu (03:08):
Yeah, no, science is certainly expensive. I met Patrick originally through Silvana actually. They actually met, so funny trivia, all three Arc founders did high school science together. Patrick and Silvana originally met in the European version of the European Young Scientist competition in high school. And Silvana and I met during our PhDs in her case at MIT and I was at Harvard, but we met at the Broad Institute sort of also a collaborative Harvard, MIT and Harvard hospitals Institute based in Kendall Square. And so, we sort of in various pairwise combinations known each other for decades and worked together for decades and have all collectively been really excited about science and technology and its potential to accelerate societal progress. Yet we also felt in our own ways that despite a lot of the tremendous progress, the structures in which we do this work, fund it, incentivize it and roll it out into the real world, seems like it's really possible that we'll undershoot that potential. And if you take 15 years ago, we didn't have the modern transformer that launched the current AI revolution, CRISPR technology, single-cell, mRNA technology or broadly addressable LNPs. That’s a tremendous amount of technologies have developed in the next 15 years. We think there's a real unique opportunity for new institutes in the 2020s to take advantage of all of these breakthroughs and the new ones that are coming to continue to accelerate biological progress but do so in a way that's fast and flexible and really focused.
Eric Topol (04:58):
Yeah, I did want to talk with you a bit. First of all before I get to the next related topic, I get a kick out of you saying you've worked or known each other for decades because I think you're only in your early thirties. Is that right?
Patrick Hsu (05:14):
I was lucky to get an early start. I first started doing research at the local university when I was 14 actually, and I was homeschooled actually until college. And so, one of the funny things that you got to do when you're homeschooled is well, you could do whatever you want. And in my case that was work in the lab. And so, I actually worked basically full time as an intern volunteer, cut my teeth in single cell patch clamp, molecular biology, protein biochemistry, two photon and focal imaging and kind of spiraled from there. I loved the lab, I loved doing bench work. It was much more exciting to me than programming computers, which was what I was doing at the time. And I think these sort of two loves have kind of brought me and us to where we are today.
Eric Topol (06:07):
Before you got to Berkeley and Arc, I know you were at Broad Institute, but did you also pick up formal training in computer science and AI or is that something that was just part of the flow?
Patrick Hsu (06:24):
So I grew up coding. I used to work through problems sets before dinner growing up. And so, it's just something that you kind of learn natively just like learning French or Mandarin.
New Models of Funding Life Science
Eric Topol (06:42):
That's what I figured. Okay. Now this model of Arc Institute came along in a kind of similar timeframe as the Arena BioWorks in Boston, where some of the faculty left to go to Arena like my friend Stuart Schreiber and many others. And then of course Priscilla and Mark formed the Chan Zuckerberg Institute and its biohub and its support. So can you contrast for one, these three different models because they’re both very different than of course the traditional NIH pathway, how Arc is similar or different to the others, and obviously the goal here is accelerating things that are going to really make a difference.
Patrick Hsu (07:26):
Yeah, the first thing I would say is zooming out. There have been lots of efforts to experiment with how we do science, the practice of science itself. And in fact, I've recently been reading this book, the Demon Under the Microscope about the history of infectious disease, and it talks about how in the 1910s through the 1930s, these German industrial dye manufacturing companies like Bayer and BASF actually launched what became essentially an early model for industrial scale science, where they were trying to develop Prontosil, Salvarsan and some of these early anti-infectives that targeted streptococcus. And these were some of the major breakthroughs that led to huge medical advances on tackling infectious disease compared to the more academic university bound model. So these trends of industrial versus academic labs and different structures to optimize breakthroughs and applications has been a through current throughout international science for the last century.
(08:38):
And so, the way that we do research today, and that's some of our core tenets at Arc is basically it hasn't always been this way. It doesn't need to necessarily be this way. And so, I think organizational experiments should really matter. And so, there's CZI, Altos, Arena, Calico, a variety of other organizational experiments and similarly we had MRC and Bell Labs and Xerox PARCS, NIBRT, GNF, Google Research, and so on. And so, I think there are lots of different ways that you can organize folks. I think at a high level you can think about ways that you can play with for-profit versus nonprofit structures. Whether you want to be a completely independent organization or if you want to be partnered with universities. If you want to be doing application driven science or really blue sky curiosity driven work. And I think also thinking through internally the types of expertise that you bring together.
(09:42):
You can think of it like a cancer institute maybe as a very vertically integrated model. You have folks working on all kinds of different areas surrounding oncology or immunotherapy and you might call that the Tower of Babel model. The other way that folks have built institutes, you might call the lily pad model where you have coverage of as many areas of biomedical research as possible. Places like the Whitehead or Salk, it will be very broad. You'll have planned epigenetics, folks looking at RNA structural biology, people studying yeast cell cycle, folks doing in vivo melanoma models. It's very broad and I think what we try to do at Arc is think about a model that you might liken more to overlapping Viking shields where there's sort of five core areas that we're deeply investing in, in genetics and genomics, computation, neuroscience, immunology and chemical biology. Now we really think of these as five areas that are maybe the minimal critical mass that you would need to make a dent on something as complicated as complex human diseases. It's certainly not the only thing that you need, but we needed a critical mass of investigators working at least in these areas.
Eric Topol (11:05):
Well, yeah, and they really converge on where the hottest advances are being made these days. Now can you work at Arc Institute without being one of these three universities or is it really that you maintain your faculty and your part of this other entity?
Patrick Hsu (11:24):
So we have a few elements to even just the academic side of the house. We have our core investigators. I'm one of them, where we have dually appointed faculty who retain their latter rank or tenured appointment in their home department, but their labs are physically cited at the Arc headquarters where we built out a lab in Stanford Research Park in Palo Alto. And so, folks move their labs there. They continue to train graduate students based on whatever graduate programs they're formally affiliated with through their university affiliation. And so, we have nearly 40 PhD students across our labs that are training on site every day.
(12:03):
So in addition to our core investigators, we also have what we call our innovation investigators, which is more of a grant program to faculty at our partner universities. They receive unrestricted funding from us to seed a new project or accelerate an existing area in their group and their labs stay at their home campus and they just get that funding to augment their work. The third way is our technical staff model where folks basically just come work at Arc and many of them also are establishing their own research groups focusing on technology R&D areas. And so, we have five of those technology centers working in molecular engineering, multi-omics, complex cellular models, in vivo models, and in machine learning.
Discovery of Bridge RNAs
Eric Topol (12:54):
Yeah, that's a great structure. In fact, just a few months ago, Patrick Collison, the other Patrick came to Stanford HAI where I'm on the board and you've summarized it really well and it's very different than the other models and other entities, companies included that you mentioned. It's really very impressive. Now speaking of impressive on June 26, this past few months ago, which incidentally is coincident with the draft genome in the year 2000, the human sequence. You and your colleagues, perhaps the most impressive jump in terms of an Arc Institute contribution published two papers back-to-back in Nature about bridge RNA: [Bridge RNAs direct programmable recombination of target and donor DNA] and [Structural mechanism of bridge RNA-guided recombination.] And before I get you to describe this breakthrough in genome editing, some would call it genome editing 3.0 or CRISPR 3.0, whatever. But what we have today in the clinic with the approval of CRISPR 1.0 for sickle cell and thalassemia is actually quite crude. I think most people will know it's just a double stranded DNA cleavage with all sorts of issues about repair and it's not very precise. And so, CRISPR 2.0 is supposed to be represented by David Liu's contributions and his efforts at Broad like prime and base editing and then comes yours. So maybe you can tell us about it and how it is has to be viewed as quite an important advance.
Patrick Hsu (14:39):
The first thing I would say before CRISPR, is that we had RNA interference. And so, even before this modern genome editing revolution with programmable CRISPRs, we had this technology that had a lot of the core selling points as well. Any target will now become druggable to us. We simply need to reprogram a guide RNA and we can get genetic access to things that are intracellular. And I think both the discovery of RNA interference by Craig Mello and Andy Fire or the invention or discovery of programmable CRISPR technologies, both depend on the same fundamental biological mechanism. These non-coding guide RNAs that are essentially a short RNA search string that you can easily reprogram to retarget a desired enzyme function, and natively both RNAi and CRISPR are molecular scissors. Their RNA or DNA nucleases that can be reprogrammed to different regions of the genome or the transcriptome to make a cut.
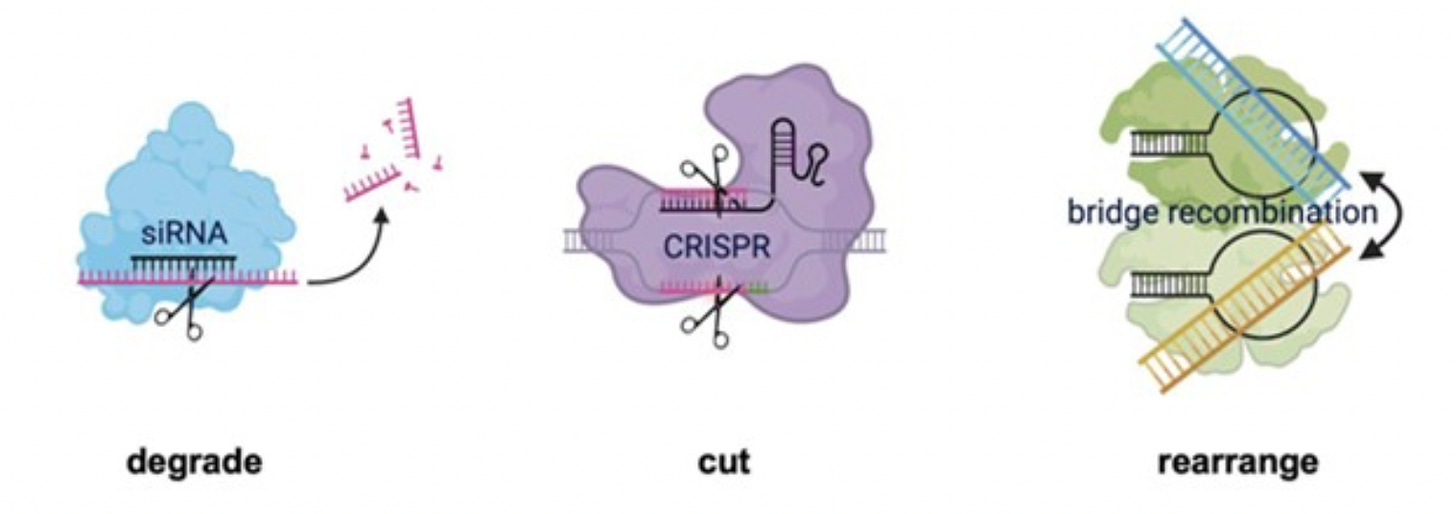
(15:48):
And as bioengineers, we have come up with all kinds of creative ways to leverage the ability to make site specific cuts to do all kinds of incredible things including genome editing or beyond transcriptional up or down regulation, molecular imaging and so on and so forth. And so, the first thing that we started thinking about in our lab was, why would mother nature have stopped only RNAi and CRISPR? There probably are lots of other non-coding RNAs out there that might be able to be programmable and if they did exist, they probably also do more complicated and interesting things than just guide a molecular scissors. So that was sort of the first core kind of intuition that we had. The second intuition that we had on the technology side, I was just wearing my biology hat, I’ll put on my technology hat, is the thing that we call genome editing today hardly involves the genome.
(16:50):
It's really you're making a cut to change an individual base or an individual gene or locus. So really you're doing small scale single locus editing, so you might call it gene level or locus level cuts. And what you really want to be able to do is do things at the genome scale at 100 kb, a megabase at the chromosome scale. And I think that's where I think the field will inevitably go if you follow the technology curves of longer and longer range gene sequencing, longer and longer range gene synthesis, and then longer and longer range gene editing. And so, what would that look like? And we started thinking, could there be essentially recombination technologies that allow you to do cut and paste in a single step. Now, the reason for that is the way that we do gene editing today involves a cut and then a multi-step process of cellular DNA repair that resolves the cut to make the exertion or the error prone deletion or the modification that ends up happening.
(17:59):
And so, it's very complicated and whether that's nucleases or base or prime editing, you're all generally limited to the small-scale single locus changes. However, there are natural mechanisms that have solved this cut and paste problem, right? There are these viruses or bacterial versions of viruses known as phage that have generally been trying to exert their multi kilobase genomes into bacterial hosts and specialize throughout billions of years. So our core thought was, well, if there are these new non-coding RNAs, what kind of functions would we be excited about? Can we look in these mobile genetic elements, these so-called jumping genes for new mechanisms? They're incredibly widespread. Transposons are thought to be some of the most diverse enzyme mechanisms found in nature. And so, we started computationally by asking ourselves a very simple question. If a mobile element inserts itself into foreign DNA and it's able to somehow be programmable, presumably the inside or something encoded in the inside of the element is predictive of some sequence on the outside of the element.
(19:15):
And so, that was the core insight we took, and we thought let's look across the boundaries of many different mobile genetic elements and we zoomed in on a particular sub family of these MGE known as insertion sequence (IS) elements which are the most autonomous minimal transposons. Normally transposons have all kinds of genes that they use to hitchhike around the genomic galaxy and endow the bacterial host with some fitness advantage like some ability to metabolize some copper and some host or some metal. And these IS elements have only the enzymes that they need to jump around. And if you identify the boundaries of these using modern computational methods, this is actually a really non-trivial problem. But if you solve that problem to figure out with nucleotide resolution where the element boundaries end and then you look for the open reading frame of the transposases enzyme inside of this element, you'll find that it's not just that coding sequence.
(20:19):
There are also these non-coding flanks inside of the element boundaries. And when we looked across the non-coding, the entire IS family tree, there are hundreds of these different types of elements. We found that this particular family IS110, had the longest non-coding ends of all IS elements. And we started doing experiments in the lab to try to figure out how these work. And what we found was that these elements are cut and paste elements, so they excise themselves into a circular form and paste themselves back in into a target site linearly. But the circularization of this element brings together two distal ends together, which brings together a -35 and a -10 box that create and reconstitute a canonical bacterial transcriptional promoter. This essentially is like plugging a plug into an electrical socket in the wall and it jacks up transcription. Now you would think this transcription would turn on the transposase enzyme so it can jump around more but it transcribes a non-coding RNA out of this non-coding end.
(21:30):
We're like, holy crap, are these RNAs actually involved in regulating the transposon? Now the boring answer would be, oh, it regulates the expression. It's like an antisense regulate or something. The exciting answer would be, oh, it's a new type of guide RNA and you found an RNA guided integrase. So we started zooming in bound dramatically on this and we undertook a covariation analysis where we were able to show that this cryptic non-coding RNA has a totally novel guide RNA structure, totally distinct from RNAi or CRISPR guide RNAs. And it had a target site that covaried with the target site of the element. And so we're like, oh wow, this could be a programmable transposase. The second thing that we found was even more surprising, there was a second region of complementarity in that same RNA that recognized the donor sequence, which is the circularized element itself. And so, this was the first example of a bispecific guide RNA, and also the first example of RNA guided self-recognition by a mobile genetic element.
Eric Topol (22:39):
It's pretty extraordinary because basically you did a systematic assessment of jumping genes or transposons and you found that they contain things that previously were not at all recognized. And then you have a way to program these to edit, change the genome without having to do any cuts or nicks, right?
Patrick Hsu (23:05):
Yeah. So what we showed in a test tube is when we took this, so-called bridge RNA, which we named because it bridges the target and donor together along with the recombinase enzyme. So the two component system, those are the only two things that you need. They're able to cut and paste DNA and recombine them in a test tube without any DNA repair, meaning that it's independent of cellular DNA repair and it does strand nicking, exchange, junction resolution and religation all in a single mechanism. So that's when we got super excited about its potential applications as bioengineering tool.
Eric Topol (23:46):
Yeah, it's pretty extraordinary. And have you already gone into in vivo assessment?
Patrick Hsu (23:54):
Yes, in our initial set of papers, what we showed is that these are programmable and functional or recombinases in a test tube and in bacterial cells. And by reprogramming the target and donor the right way, you can use these enzymes not just for insertion, but also for flipping and cutting out DNA. And so, we actually have in a single mechanism the ability to do bridge editing, if you will, for universal DNA recombination, insertion, excision or inversion, similar to what folks have been doing for decades with Cre recombinase, but with fully programmable recognition sequences. The work that we're doing now in the lab as you can imagine is to adapt these into robust tools for mammalian genome editing, including of course, human genomes. We're excited about this, we're making good progress. The CRISPR has had thousands of labs over the last 10, 15 years working on it to make these therapeutic level potency and selectivity. We're going to work and follow that same blueprint for getting bridge systems to get to that level of performance, but we're on the path and we're very optimistic for the future.
Exemplar of Digital Biology
Eric Topol (25:13):
Yeah, I think it's quite extraordinary and it's a whole different look to what we've been seeing in the CRISPR era for over the past decade and how that's been advancing and getting more specific and less need for repair and being able to be more versatile. But this takes it to yet another dimension. Now, this brings me to the field that when I think of this term digital biology, I think of you and now our mutual acquaintance, Jensen Huang, who everybody knows now. Back some months ago, he wrote and said at a conference, “Where do I think the next amazing revolution is going to come? And this is going to be flat out one of the biggest ones ever. There’s no question that digital biology is going to be it. For the first time in human history, biology has the opportunity to be engineering, not science.” So can you critique Jensen? Is he right? And tell us how you conceive the field of digital biology.
Patrick Hsu (26:20):
If you look at gene therapy today, the core concepts are actually remarkably simple. They're elegant. Of course, you're missing a broken gene, you need to put it back. And that can be curative. Very simple, powerful concept. However, for complex diseases where you don't have just a single gene that goes wrong, in many cases we actually have no idea what to do. And in fact, when you're trying to put in DNA, that's over more than a gene scale. We kind of very quickly run out of ideas. Is it a CAR and a cytokine, a CAR and a cytokine and another thing? And then we're kind of out of ideas. And so, we started thinking in the lab, how can we actually design genomes where it's not just let's reduce the genome into individual Lego blocks, iGem style with promoters and different genes that we just sort of shuffle the Lego blocks around, but actually use AI to design genome sequences.
(27:29):
So to do that, we thought we would have to first of all, train a model that can learn and decode the foreign language of biology and use that in order to design sequences. And so, we sort of have been training DNA foundation models and virtual cell models at Arc, sort of a major effort of ours where the first thing that we tried was to take a variance of transformer architecture that's used to train ChatGPT from OpenAI, but instead apply this to study the next DNA token, right? Now, the interesting thing about next token prediction in English is that you can actually learn a surprising amount of information by just predicting the next word. You can learn world knowledge is the capital of Azerbaijan, is it Baku or is it London, right? Or if you're walking around in the kitchen, then the next text is, I then left the kitchen or the bathroom, right?
(28:33):
Now you're learning about spatial reasoning, and so you can also learn translation obviously. And so similarly, I think predicting the next token or the next base and DNA can lead you to learn about molecular biochemistry, is the next amino acid residue, hydrophobic or hydrophilic. And it can teach you about the mechanics of some catalytic binding pocket or something. You can learn about a disease mutation. Is the next base, the sick linked base or the wild type base and so on and so forth. And what we found was that at massive scale, DNA foundation models learn about molecular function, not just at the DNA level, but also at the RNA and the protein. And indeed, we could use these to design molecular systems like CRISPR-Cas systems, where you have a protein and the guide RNA. It could also design new DNA transposons, and we could design sequences that look plausibly like real genomes, where we generate a megabase a million bases of continuous genome sequence. And it really looks and feels like it could be a blurry picture of something that you would actually sequence. This has been a wonderful collaboration with Brian Hie, a PI at Stanford and an Arc investigator, and we're really excited about what we've seen in this work because it promises the better performance with even more scale. And so, simply by scaling up these models, by adding in more compute, more training data or more powerful models, they're going to get sharper and sharper.
New A.I. Models in Life Science
Eric Topol (30:25):
Yeah. Well, this whole use of large language models for the language of life, whether it's the genome proteins and on and on, actually RNA and even cells has really taken root. And of course, this is really one of the foundations of that field of digital biology, which brings together generative AI, AI tools and trying to push forward our understanding in biology. And also, obviously what's been emphasized in drug discovery, perhaps it's been emphasized even too much because we still have a lot to learn about biology, but that gets me to these models. Like today, AlphaProteo was announced by DeepMind, as we all know, AlphaFold 1, 2, now 3. They were kind of precursors of being able to predict proteins from amino acid 3D structure. And that kind of took the field by a little bit like ChatGPT for life science, but now it's a new model all the time. So you've been working on various models and Arc Institute, how do you see this unfolding? Are we just going to have every aspect of the language of life being approached in all the different interactions? And this is going to help us get to a much more deep level of understanding.
Patrick Hsu (31:56):
I'll say two things. The first is a lot of models that you just described are what I would call task specific models. A model for de novo design of a binder, a model for protein structure prediction. And there are other models for protein fitness or for RNA structure prediction, et cetera, et cetera. And I think what we're going to move towards are more unifying models where there's different classes of models at different levels of scale. So we will have these atomic level models for looking at generative chemistry or ligand docking. We have models that can unify genomes and their molecules, and then we have models that can unify cells and tissues. And so, for example, if you took an H&E stain of some liver, there are folks building models where you can then predict what the single cell spatial transcriptome will look like of that model. And that's obviously operating at a very different level of abstraction than a de novo protein binder. But in the long run, all of these are going to get, I think unified. I think the reason why this is possible is that biology, unlike physics, actually has this unifying theory of evolution that runs across all of its length scales from atomic, molecular, cellular, organismal to entire ecosystem. And the promise of these models is no short then to make biology a predictive discipline.
Patrick Hsu (33:37):
In physics, the experimentalists win the big prizes for the theorists when they measure gravitational waves or whatever. But in biology, we're very practical people. You do something three times and do a T-test. And I think my prediction is we can actually gauge the success of these LLMs or whatever in biology by how much we respect theory in this field.
The A.I. Scientist
Eric Topol (34:05):
Yeah. Well, that's a really interesting perspective, an important perspective because the proliferation of models, which we're going to get into not just doing the things that you described, but also being able to be “pseudo” scientists, the so-called AI scientist. Maybe you could comment about that concept because that's been the idea that everything from the question that could be asked to the hypothesis and the experiment design and the analysis of data and then the feedback. So what is the role of the scientists, that seems to have been overplayed? And maybe you can put that in context.
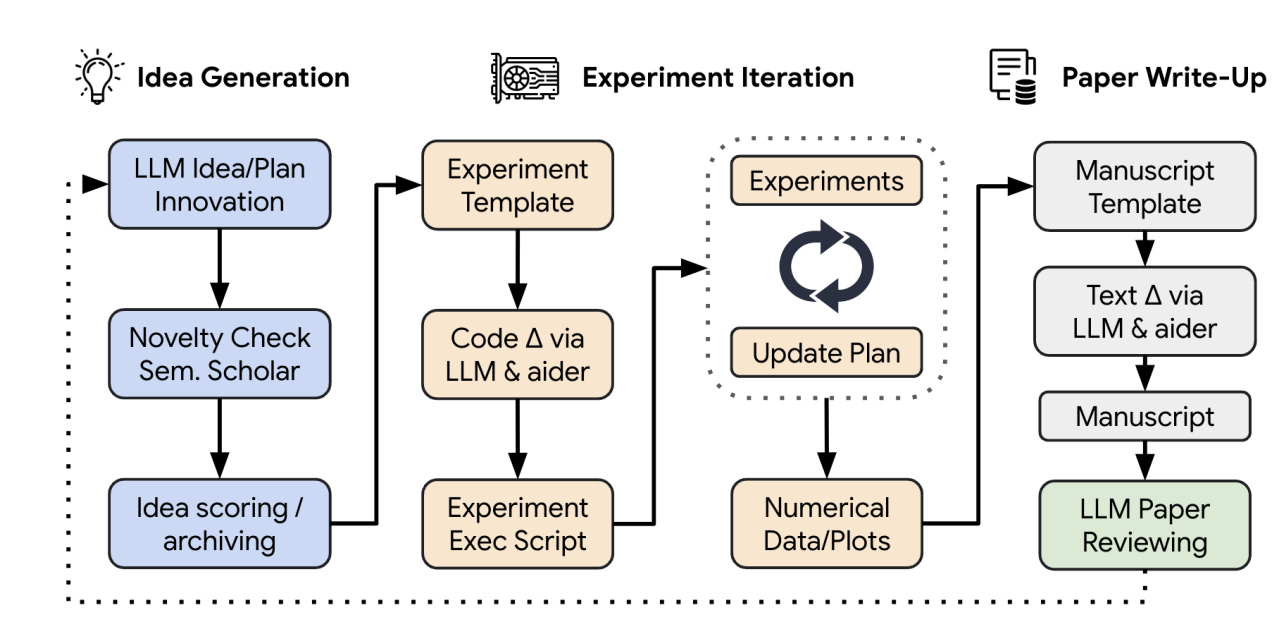
Patrick Hsu (34:48):
So yeah, right now there's a lot of excitement that we can use AI agents not just to do software enterprise workflows, but to be a research assistant. And then over time, itself an autonomous research scientist that can read the literature, come up with an idea, maybe run a bunch of robots in the lab or do a bunch of computational analyses and then potentially even analyze data, conclude what is going on and actually write an entire paper. Now, I think the vision of this is compelling in the long term. I think the question is really about timescale. If you break down the scientific method into its constituent parts, like hypothesis generation, doing an experiment, analyzing experiment and iterating, we're clearly going to use AI of some kind at every single step of this cycle. I think different steps will require different levels of maturity. The way that I would liken this is just wet lab automation, folks have dreamed about having pipetting robots that just do their western blots and do their cell culture for them for generations.
(36:01):
But of course, today they don't actually really feel fundamentally different from the same ones that we had in the 90s, let's say. Right? And so, obviously they're getting better, but it seems to me one of the trends I'm very bullish about is the explosion of humanoid robots and robot foundation models that have a world model and a sense of physics and proportionate space loaded onto them. Within five years, we're going to have home robots that can fold your clothes, that can organize your kitchen and do all of this while you're sleeping, so you wake up to a clean home every day.
Eric Topol (36:40):
It’s not going to be just Roomba anymore. There's going to be a lot more, but it isn't just the hardware, it's also the agents playing in software, right?
Patrick Hsu (36:50):
It's the integrated loop of the hardware and the software where the ability to make the same machine generally intelligent will make it adaptable to a broad array of tasks. Now, what I'm excited about is those generally intelligent humanoid robots coming into the lab, where instead of creating a centrifuge or a new type of pipetter that's optimized for your Beckman or Hamilton device, instead you just have robot arms that you snap onto the edge of the bench and then they just work alongside you. And I do think that's coming, although it'll take a lot of hardware and software and computer vision engineering to make that possible.
A Sense of Humor
Eric Topol (37:32):
Yeah, and I think also going back to originating the question, there still is quite a debate about the creativity and the lack of any simulation of AGI, whatever that means anymore. And so, the human in the loop part of this is obviously I think it's still of critical nature. Now, the other thing I learned about you is you have a great sense of humor, which is really important by the way. And recently, which is great that you're active on X or Twitter because that's one way we get to see what you're thinking on a day-to-day basis. But I think you put out a poll which was really quite provocative , and it was about, here's what it said, “do more people in the world *truly* understand transformers or health insurance?” And interestingly, you got 49% for transformers at 51% for health insurance. Can you tell us what you're thinking when you put that poll together? Because obviously a lot of people don't understand either of these.
Patrick Hsu (38:44):
I think the core question is, there are different ways of looking at the world, some of which are very bottom up and some of which are very top down. And one of the very surprising things about transformers is they're taking something that is in principle, an incredibly simple task, which is if you have a string of text, what is the next letter? And somehow at massive, massive scale, you can unlock something that looks an awful lot like reasoning, and you've got these emergent behaviors. Now the bottoms up theory of just the linear algebra that's going on in these models couldn't possibly really help us predict that we have these emerging capabilities. And I think similarly in healthcare, there's a literal set of parts that are operating in some complex way that at massive scale becomes this incredibly confusing and dynamic system for how we can actually incentivize how we make medicines, how we actually take care of people, and how we actually pay for any of this from an economic point of view. And so, I think it was, in some sense if transformers can actually be an explainable by just linear algebra equations, maybe there will be a way to decompose the seemingly incredibly confusing world of healthcare in order to actually build a better way forward.
Computing Power and the GPU Arms Race
Eric Topol (40:12):
Yeah. Well that's great. Now the other thing I wanted to ask you about, we open source and the arms race of GPUs and this whole kind of idea is you touched on the need for coalescing a lot of these tools to exploit the synergy. But we have an issue because many academic labs like here at Scripps Research and so many others, including as I learned even at Stanford, have limited access to GPUs. So computing power of large language models is a problem. And then the models that exist today that can be adopted like Llama or others, and they're somewhat limited. And then we also have a movement towards trying to make things more open source, like for example, recently OpenCRISPR with Profluent Bio that is basically trying to use AI for CRISPR guides. And so, how do you deal with this arms race, computing power, open source, proprietary models that are not easily accessible without a lot of resources?
Patrick Hsu (41:30):
So the first thing I would say is, we are in the academic science sphere really unprepared for the level of resources that are required for doing this type of cutting edge computational work. There are top Stanford computer science professors or computational researchers who have a single GPU in their office, and that's actually what their whole lab runs off of.
(41:58):
The UC Berkeley campus, the grid runs on something like 12 megawatts of power and how are they going to build an on-premises GPU clusters, like a central question that can scale across the entire needs? And these are two of the top computer science universities in the world. And so, I think one of our kind of core beliefs at Arc is, as science both experimentally and computationally has gotten incredibly complex, not just in terms of conceptually, but also just the actual infrastructure and machines and know-how that you need to do things. We actually need to essentially support this. So we have a private GPU cloud that we use to train our models, and we have access to significantly large clusters for large burst kind of train outs as necessary. And I think infrastructurally for running genomics experiments or doing scalable brain organoid screens, right, we're also building out the infrastructure to support that experimentally.
Eric Topol (43:01):
Yeah, no, I think this is one of the advantages of the new model like the Arc Institute because not many centers have that type of plasticity with access to computing power when needed. So that's where a brilliant mind you and the Arc Institute together makes for a formidable recipe for future advances and of course building on the ones you've already accomplished.
The Primacy of Human Talent
Patrick Hsu (43:35):
I would just say, my main skill, if I have one, is to recruit really, really smart people. And so, everything that you're seeing and hearing about is the work of unbelievable colleagues who are curious, passionate, and incredible scientists.
Eric Topol (43:53):
But it also takes the person who can judge those who are in that category set as a role model. And you're certainly doing that. I guess just in closing, I mean, it's just such a delight to get to meet you here and kind of get your thoughts on what is the hottest thing in life science without question, which brings together the fields of AI and what's going on, not just obviously in genome editing, but this digital biology era that we're still in the early phases of, I mean, I think you could say that it's just going to continue to accelerate the exponential curve. We're still kind of on the bottom of that, I would imagine where we're headed. Any other things that you want to bring up that I haven't touched on that will round out this conversation?
Patrick Hsu (44:50):
I mean, I think it's very early days here at Arc.
Patrick Hsu (44:53):
When we founded Arc, we asked ourselves, how do we measure success? We don't have customers or revenue in the way that a typical startup does. And we felt sort of three things. The first was research institutes live and die by their talent. Can we actually hire incredible people when we make offers to people we want to come, do they come? The second was, when those folks do come to Arc, do they feel like they're able to work on important research programs that they couldn't do sort of at their prior university or company? And then longer term, the third thing was, and there's just no shortcut around this, you need to do important work. And I think we've been really excited that there are early signs that we're able to do all three of these things, and we're still, again, just following the same scaling laws that we're seeing in natural language and vision, but for the domain of biology. And so, we're excited about what's ahead and think if there are folks who are interested in learning more about Arc, just shoot me an email or DM.
Eric Topol (46:07):
Yeah, well I would just say, congratulations on what you've already achieved. I know you're going to keep rocking it because you already have in a short time. And for anybody who doesn't know about Arc Institute and your work and your team, I hope this is going to be putting them on notice actually what can be accomplished outside of the usual NIH funded model, which is kind of a risk-free zone where you basically have to have your results nailed down before you send in your proposal frequently, and it doesn't do great things for young people. Really, I think you actually qualify in that demographic where it's hard for them to break in for getting NIH grants and also for this type of work that you're doing. So we'll look for the next bridge beyond bridge RNAs of your just fantastic efforts. So Patrick, thanks so much for joining us today, and we'll be checking back with you and following all the great work that you'll be doing in the times ahead.
Patrick Hsu (47:14):
Thanks so much, Eric. It was such a pleasure to be here today. Appreciate the opportunity.
*******************
Thanks for listening, reading or watching!
The Ground Truths newsletters and podcasts are all free, open-access, without ads.
Please share this post/podcast with your friends and network if you found it informative!
Voluntary paid subscriptions all go to support Scripps Research. Many thanks for that—they greatly help fund our summer internship programs.
Thanks to my producer Jessica Nguyen and Sinjun Balabanoff for audio and video support at Scripps Research.
Note: you can select preferences to receive emails about newsletters, podcasts, or all I don’t want to bother you with an email for content that you’re not interested in.
Patrick Hsu: A Trailblazer in Digital Biology